A Review of Artificial Intelligence Tools for the Management of Cleaning and Tracking in Photovoltaic Systems
Keywords:
Cleaning, tracking, soiling, photovoltaic system, machine learning, artificial intelligence, solar.Abstract
Solar Photovoltaic System (SPV) is a rapidly expanding renewable energy source integrated into national power grids and off-grid systems worldwide. Therefore, the effective management of power systems and the energy industry needs accurate solar energy forecasting, ranging from short-term predictions of a few seconds to longer-term forecasts up to one week in advance. Hence it is well recognized that the presence of dust particles on the surface of photovoltaic (PV) modules substantially influences their overall performance. Subsequently, the resultant decrease in energy generation significantly impacts the financial earnings. A range of methodologies have been used to address the limitations associated with solar radiation forecasting. The use of machine learning (ML) algorithms provides a range of techniques for predicting solar radiation. Additionally, integrating artificial intelligence (AI) and ML may collectively enhance the optimization of cleaning intervals for photovoltaic (PV) modules. In the same manner, the development of a sun tracking algorithm aims to accurately determine the location of the sun in order to optimize the reception of solar energy. This article aims to critically review the current research on maintenance and tracking strategies for PV panels while presenting a novel application of AI and ML algorithms. Finally, this review presents a comprehensive analysis comparing traditional approaches with AI techniques to demonstrate the significant impact of AI algorithms in PV systems.
References
Abdulla, H.; Sleptchenko, A. and Nayfeh, A. (2024). Photovoltaic systems operation and maintenance: A review and future directions. Renewable and Sustainable Energy Reviews, 195, 114342.
Abubakar, A.; Almeida, C. F. M. and Gemignani, M. (2021). Review of artificial intelligence-based failure detection and diagnosis methods for solar photovoltaic systems. Machines, 9, 328.
Ahmad, E. Z.; Jarimi, H. and Razak, T. R. (2022). Artificial Neural Network Prediction Model of Dust Effect on Photovoltaic Performance for Residential Applications: Malaysia Case Study. Int. J. Renew. En. Dev, 11, 365-373.
Alghazo, J.; Latif, G.; Hammad, B.; Al-Abed, M.; Sibai, F. and Al-Kouz, W. (2023). Prediction of the Performance of a Sun Tracking Photovoltaic System using different Artificial Intelligence Techniques: Case Study in Zarqa, Jordan. International Journal of Computing and Digital Systems, 13, 1-1.
Alvarez, D. L.; Al-Sumaiti, A. S. and Rivera, S. R. (2020). Estimation of an optimal PV panel cleaning strategy based on both annual radiation profile and module degradation. IEEE Access, 8, 63832-63839.
Appels, R.; Muthirayan, B.; Beerten, A.; Paesen, R.; Driesen, J. and Poortmans, J. (2012). The effect of dust deposition on photovoltaic modules. 2012 38th IEEE photovoltaic specialists conference. IEEE, 001886-001889.
Babay, M.-A.; Adar, M.; Touairi, S.; Chebak, A. and Mabrouki, M. (2024). Numerical Simulation and Thermal Analysis of Pressurized Hydrogen Vehicle Cylinders: Impact of Geometry and Phase Change Materials. Journal of Advanced Research in Fluid Mechanics and Thermal Sciences, 117, 71-90.
Barbieri, F.; Rajakaruna, S. and Ghosh, A. (2017). Very short-term photovoltaic power forecasting with cloud modeling: A review. Renewable and Sustainable Energy Reviews, 75, 242-263.
Carballo, J. A.; Bonilla, J.; Berenguel, M.; Fernández-Reche, J. and García, G. (2019). Machine learning for solar trackers. AIP Conference Proceedings. AIP Publishing.
Chaichan, M. T.; Mohammed, B. A. and Kazem, H. A. (2015). Effect of pollution and cleaning on photovoltaic performance based on experimental study. International Journal of Scientific and Engineering Research, 6, 594-601.
Chiteka, K.; Arora, R.; Sridhara, S. and Enweremadu, C. (2020). A novel approach to Solar PV cleaning frequency optimization for soiling mitigation. Scientific African, 8, e00459.
Chowdhury, M. E.; Khandakar, A.; Hossain, B. and Abouhasera, R. (2019). A low-cost closed-loop solar tracking system based on the sun position algorithm. Journal of Sensors, 2019.
Darwish, Z. A.; Kazem, H. A.; Sopian, K.; Al-Goul, M. and Alawadhi, H. (2015). Effect of dust pollutant type on photovoltaic performance. Renewable and Sustainable Energy Reviews, 41, 735-744.
Darwish, Z. A.; Kazem, H. A.; Sopian, K.; Alghoul, M. and Chaichan, M. T. (2013). Impact of some environmental variables with dust on solar photovoltaic (PV) performance: review and research status. International J of Energy and Environment, 7, 152-159.
Darwish, Z. A.; Sopian, K. and Fudholi, A. (2021). Reduced output of photovoltaic modules due to different types of dust particles. Journal of Cleaner Production, 280, 124317.
Derakhshandeh, J. F.; Alluqman, R.; Mohammad, S.; Alhussain, H.; Alhendi, G.; Aleid, D. and Ahmad, Z. (2021). A comprehensive review of automatic cleaning systems of solar panels. Sustainable Energy Technologies and Assessments, 47, 101518.
Edenhofer, O.; Pichs-Madruga, R.; Sokona, Y.; Seyboth, K.; Kadner, S.; Zwickel, T.; Eickemeier, P.; Hansen, G.; Schlömer, S. and Von Stechow, C. (2011). Renewable energy sources and climate change mitigation: Special report of the intergovernmental panel on climate change, Cambridge University Press.
Fan, Z.; Wang, M.; Mu, J. and Yi, J. (2020). Alternative cleaning and dust detection method for PV modules and its application. Journal of Renewable and Sustainable Energy, 12.
Fathabadi, H. (2016). Novel high efficient offline sensorless dual-axis solar tracker for using in photovoltaic systems and solar concentrators. Renewable Energy, 95, 485-494.
Feng, H. (2018). The application of artificial intelligence in electrical automation control. Journal of Physics: Conference Series. IOP Publishing, 062008.
Frizzo Stefenon, S.; Kasburg, C.; Nied, A.; Rodrigues Klaar, A. C.; Silva Ferreira, F. C. and Waldrigues Branco, N. (2020). Hybrid deep learning for power generation forecasting in active solar trackers. IET Generation, Transmission & Distribution, 14, 5667-5674.
Hammad, B.; Al–Abed, M.; Al–Ghandoor, A.; Al–Sardeah, A. and Al–Bashir, A. (2018). Modeling and analysis of dust and temperature effects on photovoltaic systems’ performance and optimal cleaning frequency: Jordan case study. Renewable and Sustainable Energy Reviews, 82, 2218-2234.
Harrou, F.; Dairi, A.; Taghezouit, B. and Sun, Y. (2019). An unsupervised monitoring procedure for detecting anomalies in photovoltaic systems using a one-class support vector machine. Solar Energy, 179, 48-58.
Hasan, N. M. S.; Sobuz, M. H. R.; Shaurdho, N. M. N.; Meraz, M. M.; Datta, S. D.; Aditto, F. S.; Kabbo, M. K. I. and Miah, M. J. (2023). Eco-friendly concrete incorporating palm oil fuel ash: Fresh and mechanical properties with machine learning prediction, and sustainability assessment. Heliyon, 9.
He, K.; Gkioxari, G.; Dollár, P. and Girshick, R. (2017). Mask r-cnn. Proceedings of the IEEE international conference on computer vision. 2961-2969.
Heinrich, M.; Meunier, S.; Samé, A.; Quéval, L.; Darga, A.; Oukhellou, L. and Multon, B. (2020). Detection of cleaning interventions on photovoltaic modules with machine learning. Applied Energy, 263, 114642.
Hosmer Jr, D. W.; Lemeshow, S. and Sturdivant, R. X. (2013). Applied logistic regression, John Wiley & Sons.
Howard, A. G.; Zhu, M.; Chen, B.; Kalenichenko, D.; Wang, W.; Weyand, T.; Andreetto, M. and Adam, H. (2017). Mobilenets: Efficient convolutional neural networks for mobile vision applications. arXiv preprint arXiv:1704.04861.
Irena (2023). Renewable Capacity Statistics. 2022 [Online].
Jacob, B.; Kligys, S.; Chen, B.; Zhu, M.; Tang, M.; Howard, A.; Adam, H. and Kalenichenko, D. (2018). Quantization and training of neural networks for efficient integer-arithmetic-only inference. Proceedings of the IEEE conference on computer vision and pattern recognition. 2704-2713.
Jamroen, C.; Komkum, P.; Kohsri, S.; Himananto, W.; Panupintu, S. and Unkat, S. (2020). A low-cost dual-axis solar tracking system based on digital logic design: Design and implementation. Sustainable Energy Technologies and Assessments, 37, 100618.
Javed, W.; Guo, B. and Figgis, B. (2017). Modeling of photovoltaic soiling loss as a function of environmental variables. Solar Energy, 157, 397-407.
Jiang, Y.; Lu, L. and Lu, H. (2016). A novel model to estimate the cleaning frequency for dirty solar photovoltaic (PV) modules in desert environment. Solar Energy, 140, 236-240.
Jones, R. K.; Baras, A.; Al Saeeri, A.; Al Qahtani, A.; Al Amoudi, A. O.; Al Shaya, Y.; Alodan, M. and Al-Hsaien, S. A. (2016). Optimized cleaning cost and schedule based on observed soiling conditions for photovoltaic plants in central Saudi Arabia. IEEE journal of photovoltaics, 6, 730-738.
Kalogirou, S.; Lalot, S.; Florides, G. and Desmet, B. (2008). Development of a neural network-based fault diagnostic system for solar thermal applications. Solar Energy, 82, 164-172.
Kazem, H. A.; Yousif, J.; Chaichan, M. T. and Al‐Waeli, A. H. (2019). Experimental and deep learning artificial neural network approach for evaluating grid‐connected photovoltaic systems. International Journal of Energy Research, 43, 8572-8591.
Kermadi, M. and Berkouk, E. M. (2017). Artificial intelligence-based maximum power point tracking controllers for Photovoltaic systems: Comparative study. Renewable and Sustainable Energy Reviews, 69, 369-386.
Khilar, R.; Suba, G. M.; Kumar, T. S.; Samson Isaac, J.; Shinde, S. K.; Ramya, S.; Prabhu, V. and Erko, K. G. (2022). Improving the efficiency of photovoltaic panels using machine learning approach. International Journal of Photoenergy, 2022.
Kumar, S. and Dave, V. (2016). ANN based controller to mitigate soiling loss on solar panels. 2016 21st Century Energy Needs-Materials, Systems and Applications (ICTFCEN). IEEE, 1-6.
Kurukuru, V. S. B.; Haque, A.; Khan, M. A.; Sahoo, S.; Malik, A. and Blaabjerg, F. (2021). A review on artificial intelligence applications for grid-connected solar photovoltaic systems. Energies, 14, 4690.
Lee, W.; Kim, K.; Park, J.; Kim, J. and Kim, Y. (2018). Forecasting solar power using long-short term memory and convolutional neural networks. IEEE access, 6, 73068-73080.
Li, Z.; Liu, X. and Tang, R. (2010). Optical performance of inclined south-north single-axis tracked solar panels. Energy, 35, 2511-2516.
Maghami, M. R.; Hizam, H.; Gomes, C.; Radzi, M. A.; Rezadad, M. I. and Hajighorbani, S. (2016). Power loss due to soiling on solar panel: A review. Renewable and Sustainable Energy Reviews, 59, 1307-1316.
Malav, S. and Vadhera, S. (2015). Hardware implementation of solar tracking system using a stepper motor. 2015 International Conference on Energy, Power and Environment: Towards Sustainable Growth (ICEPE). IEEE, 1-4.
Mathworks (2023a). Deep Learning Toolbox - MATLAB. [Online]..
Mathworks (2023b). Train support vector machine (SVM) classifier-MATLAB [Online].
Mohanraj, M.; Jayaraj, S. and Muraleedharan, C. (2009). Performance prediction of a direct expansion solar assisted heat pump using artificial neural networks. Applied Energy, 86, 1442-1449.
Muhammad Ehsan, R.; Simon, S. P. and Venkateswaran, P. (2017). Day-ahead forecasting of solar photovoltaic output power using multilayer perceptron. Neural Computing and Applications, 28, 3981-3992.
Munawar, M. I.; Alam, I. and Ali, M. S. (2023). An efficient energy prediction model for solar energy power system using Artificial Intelligence technique. International Conference on Recent Academic Studies. 139-145.
Naeem, M. and Tamizhmani, G. (2015). Cleaning frequency optimization for soiled photovoltaic modules. 2015 IEEE 42nd Photovoltaic Specialist Conference (PVSC). IEEE, 1-5.
Ning, C.; Zhou, H.; Song, Y. and Tang, J. (2017). Inception single-shot multibox detector for object detection. 2017 IEEE International Conference on Multimedia & Expo Workshops (ICMEW). IEEE, 549-554.
Nsengiyumva, W.; Chen, S. G.; Hu, L. and Chen, X. (2018). Recent advancements and challenges in Solar Tracking Systems (STS): A review. Renewable and Sustainable Energy Reviews, 81, 250-279.
Osmani, K.; Haddad, A.; Lemenand, T.; Castanier, B. and Ramadan, M. (2020). A review on maintenance strategies for PV systems. Science of the Total Environment, 746, 141753.
Paul, D. I. (2022). Dust Deposition on Photovoltaic Modules: Its Effects on Performance. The Effects of Dust and Heat on Photovoltaic Modules: Impacts and Solutions, 3-46.
Pavan, A. M.; Mellit, A. and De Pieri, D. (2011). The effect of soiling on energy production for large-scale photovoltaic plants. Solar energy, 85, 1128-1136.
Pavan, A. M.; Mellit, A.; De Pieri, D. and Kalogirou, S. A. (2013). A comparison between BNN and regression polynomial methods for the evaluation of the effect of soiling in large scale photovoltaic plants. Applied energy, 108, 392-401.
Pulipaka, S. and Kumar, R. (2016). Power prediction of soiled PV module with neural networks using hybrid data clustering and division techniques. Solar Energy, 133, 485-500.
Qazi, A.; Fayaz, H.; Wadi, A.; Raj, R. G.; Rahim, N. and Khan, W. A. (2015). The artificial neural network for solar radiation prediction and designing solar systems: a systematic literature review. Journal of cleaner production, 104, 1-12.
Razak, T. R.; Garibaldi, J. M.; Wagner, C.; Pourabdollah, A. and Soria, D. (2020). Toward a framework for capturing interpretability of hierarchical fuzzy systems—a participatory design approach. IEEE Transactions on Fuzzy Systems, 29, 1160-1172.
Ritchie, H. and Roser, M. (2020). Renewable Energy. Our World in Data 2020 [Online].
Robles Algarín, C.; Sevilla Hernández, D. and Restrepo Leal, D. (2018). A low-cost maximum power point tracking system based on neural network inverse model controller. Electronics, 7, 4.
Rodrigo, P. M.; Gutiérrez, S.; Micheli, L.; Fernández, E. F. and Almonacid, F. (2020). Optimum cleaning schedule of photovoltaic systems based on levelised cost of energy and case study in central Mexico. Solar Energy, 209, 11-20.
Salamah, T.; Ramahi, A.; Alamara, K.; Juaidi, A.; Abdallah, R.; Abdelkareem, M. A.; Amer, E.-C. and Olabi, A. G. (2022). Effect of dust and methods of cleaning on the performance of solar PV module for different climate regions: Comprehensive review. Science of The Total Environment, 827, 154050.
Sandler, M.; Howard, A.; Zhu, M.; Zhmoginov, A. and Chen, L.-C. (2018). Mobilenetv2: Inverted residuals and linear bottlenecks. Proceedings of the IEEE conference on computer vision and pattern recognition. 4510-4520.
Saravanan, S. and Babu, N. R. (2016). Maximum power point tracking algorithms for photovoltaic system–A review. Renewable and Sustainable Energy Reviews, 57, 192-204.
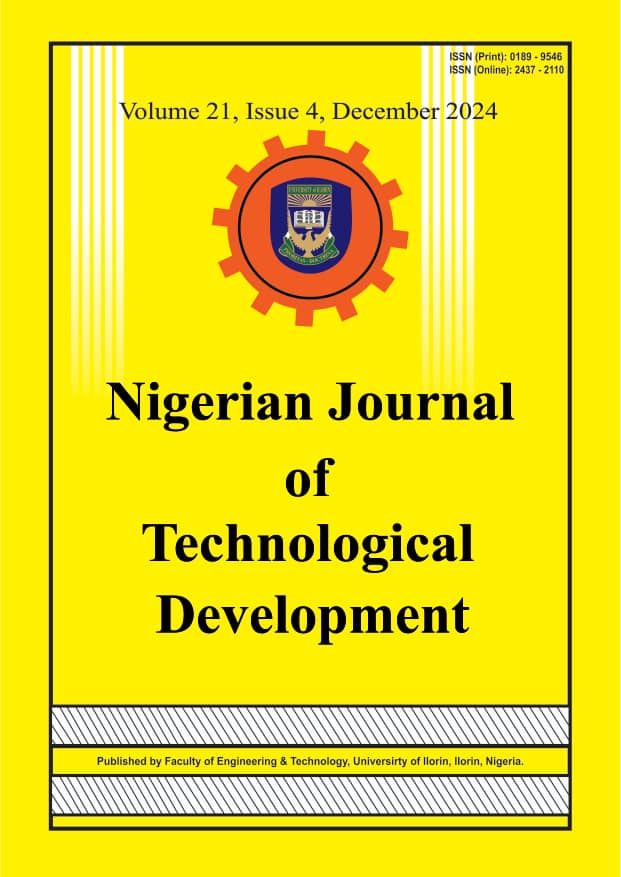
Downloads
Published
Issue
Section
License
Copyright (c) 2024 Nigerian Journal of Technological Development

This work is licensed under a Creative Commons Attribution-NonCommercial-ShareAlike 4.0 International License.
In accordance with the Copyright Act of 1976, which became effective January 1, 1978, the following statement signed by each author must accompany the manuscript submitted: "I, the undersigned author, transfer all copyright ownership of the manuscript referenced above to the Nigerian Journal of Technological Development, in the event the work is published. I warrant that the article is original, does not infringe upon any copyright or other proprietary right of any third party, is not under consideration by another journal, and has not been published previously. I have reviewed and approve the submitted version of the manuscript and agree to its publication in the Nigerian Journal of Technological Development." A copyright transfer form should be downloaded from the NJTD Website ( http://njtd.com.ng/index.php/njtd). Author(s) will be consulted, whenever possible, regarding republication of material. All authors must have access to the data presented and the authors and sponsor (if applicable) must agree to share original data with the editor if requested.