Power Quality disturbance monitoring in PV integrated power system with mode decomposition and ensemble extreme learning machine
Keywords:
Power quality, Ensemble extreme learning machine, PV integration, Variational mode decompositionAbstract
Power quality disturbance (PQD) monitoring has become an important issue in modern power system due to integration of several renewable energy sources such as photovoltaic (PV), wind energy system (WES), Fuel cells etc. This research presents a mode decomposed based ensemble extreme learning machine (EELM) to recognise and classify the PQD events with higher accuracy in terms of rapid learning speed and smaller computational burden in a complete PV based power system, The PQD signals are decomposed using a variational mode decomposition (VMD) to obtain effective band limited intrinsic mode functions (BIIMF) which leads to compute robust features and improves classification accuracy. For Power Quality Disturbances detection and classification, an ensemble extreme learning machine is suggested since an ensemble outperforms any single contributing model in terms of performance and prediction. The proposed VMD-EELM approach is validated in a modified IEEE 13 Bus system integrating PV with ten types of PQD. It is being demonstrated that the suggested method can reliably identify and track PQD occurrences both with and without noise.
References
Achlerkar, P. D., Samantaray, S. R., and Manikandan, M. S. (2016). Variational mode decomposition and decision tree based detection and classification of power quality disturbances in grid-connected distributed generation system. IEEE Transactions on Smart Grid, 9(4), 3122-3132.
Chawda, G. S., Shaik, A. G., Shaik, M., Padmanaban, S., Holm-Nielsen, J. B., Mahela, O. P., and Kaliannan, P. (2020). Comprehensive review on detection and classification of power quality disturbances in utility grid with renewable energy penetration. IEEE Access, 8, 146807-146830.
Chen, C., Li, W., Su, H.,and Liu, K. (2014). Spectral-spatial classification of hyperspectral image based on kernel extreme learning machine. Remote sensing, 6(6), 5795-5814.
Chen, Z., Jiang, C., and Xie, L. (2018). A novel ensemble ELM for human activity recognition using smartphone sensors. IEEE Transactions on Industrial Informatics, 15(5), 2691-2699.
Dalai, S., Dey, D., Chatterjee, B., Chakravorti, S., and Bhattacharya, K. (2013, December). Cross Hilbert-Huang transform based feature extraction method for multiple PQ disturbance classification. In 2013 IEEE 1st International Conference on Condition Assessment Techniques in Electrical Systems (CATCON) (pp. 314-317). IEEE.
Dragomiretskiy, K., and Zosso, D. (2013). Variational mode decomposition. IEEE transactions on signal processing, 62(3), 531-544.
Elphick, S., Ciufo, P., Smith, V., and Perera, S. (2015, September). Summary of the economic impacts of power quality on consumers. In 2015 Australasian Universities power engineering conference (AUPEC) (pp. 1-6). IEEE.
Eristi, B., and Eristi, H. (2022). Classification of Power Quality Disturbances in Solar PV Integrated Power System Based on a Hybrid Deep Learning Approach. International Transactions on Electrical Energy Systems, 2022.
Granados-Lieberman, D., Romero-Troncoso, R. J., Osornio-Rios, R. A., Garcia-Perez, A., and Cabal-Yepez, E. (2011). Techniques and methodologies for power quality analysis and disturbances classification in power systems: a review. IET Generation, Transmission & Distribution, 5(4), 519-529.
He, S., Li, K., and Zhang, M. (2013). A real-time power quality disturbances classification using hybrid method based on S-transform and dynamics. IEEE transactions on instrumentation and measurement, 62(9), 2465-2475.
Huang, G., Huang, G. B., Song, S., and You, K. (2015). Trends in extreme learning machines: A review. Neural Networks, 61, 32-48.
Kersting, W. H. (1991). Radial distribution test feeders. IEEE Transactions on Power Systems, 6(3), 975-985.
Lin, W. M., Wu, C. H., Lin, C. H., and Cheng, F. S. (2008). Detection and classification of multiple power-quality disturbances with wavelet multiclass SVM. IEEE transactions on power delivery, 23(4), 2575-2582.
Liu, H., Hussain, F., Shen, Y., Arif, S., Nazir, A., and Abubakar, M. (2018). Complex power quality disturbances classification via curvelet transform and deep learning. Electric Power Systems Research, 163, 1-9.
Liu, H., Hussain, F., Shen, Y., Arif, S., Nazir, A., and Abubakar, M. (2018). Complex power quality disturbances classification via curvelet transform and deep learning. Electric Power Systems Research, 163, 1-9.
Liu, H., Hussain, F., Shen, Y., Morales-Menendez, R., Abubakar, M., Junaid Yawar, S., and Arain, H. J. (2019). Signal processing and deep learning techniques for power quality events monitoring and classification. Electric Power Components and Systems, 47(14-15), 1332-1348.
Liu, H., Hussain, F., Shen, Y., Morales-Menendez, R., Abubakar, M., Junaid Yawar, S., and Arain, H. J. (2019). Signal processing and deep learning techniques for power quality events monitoring and classification. Electric Power Components and Systems, 47(14-15), 1332-1348.
Liu, W., Liu, Y., Li, S., and Chen, Y. (2023). A Review of Variational Mode Decomposition in Seismic Data Analysis. Surveys in Geophysics, 44(2), 323-355.
Mishra, M. (2019). Power quality disturbance detection and classification using signal processing and soft computing techniques: A comprehensive review. International transactions on electrical energy systems, 29(8), e12008.
Motlagh, S. Z., and Foroud, A. A. (2021). Power quality disturbances recognition using adaptive chirp mode pursuit and grasshopper optimized support vector machines. Measurement 168, 108461.
Shah, R., Mithulananthan, N., Bansal, R. C., and Ramachandaramurthy, V. K. (2015). A review of key power system stability challenges for large-scale PV integration. Renewable and Sustainable Energy Reviews, 41, 1423-1436.
Stefenon, S. F., Grebogi, R. B., Freire, R. Z., Nied, A., and Meyer, L. H. (2019). Optimized ensemble extreme learning machine for classification of electrical insulators conditions. IEEE transactions on industrial electronics, 67(6), 5170-5178.
Thirumala, K., Umarikar, A. C., and Jain, T. (2014). Estimation of single-phase and three-phase power-quality indices using empirical wavelet transform. IEEE Transactions on power delivery, 30(1), 445-454.
Wang, S., and Chen, H. (2019). A novel deep learning method for the classification of power quality disturbances using deep convolutional neural network. Applied energy, 235, 1126-1140.
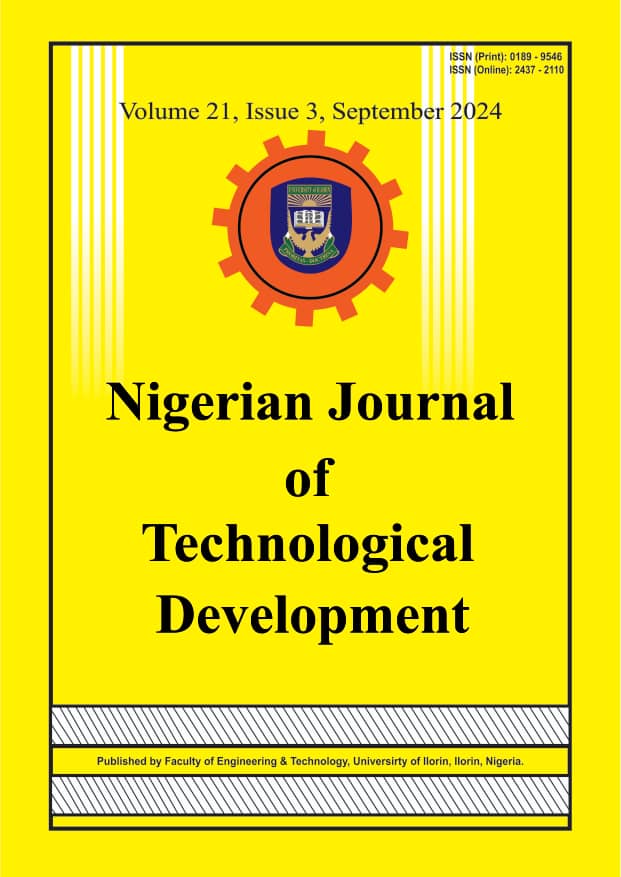
Downloads
Published
Issue
Section
License
Copyright (c) 2024 Nigerian Journal of Technological Development

This work is licensed under a Creative Commons Attribution-NonCommercial-ShareAlike 4.0 International License.
In accordance with the Copyright Act of 1976, which became effective January 1, 1978, the following statement signed by each author must accompany the manuscript submitted: "I, the undersigned author, transfer all copyright ownership of the manuscript referenced above to the Nigerian Journal of Technological Development, in the event the work is published. I warrant that the article is original, does not infringe upon any copyright or other proprietary right of any third party, is not under consideration by another journal, and has not been published previously. I have reviewed and approve the submitted version of the manuscript and agree to its publication in the Nigerian Journal of Technological Development." A copyright transfer form should be downloaded from the NJTD Website ( http://njtd.com.ng/index.php/njtd). Author(s) will be consulted, whenever possible, regarding republication of material. All authors must have access to the data presented and the authors and sponsor (if applicable) must agree to share original data with the editor if requested.